Searching for new materials
How simulations and machine learning are helping BAM to do so
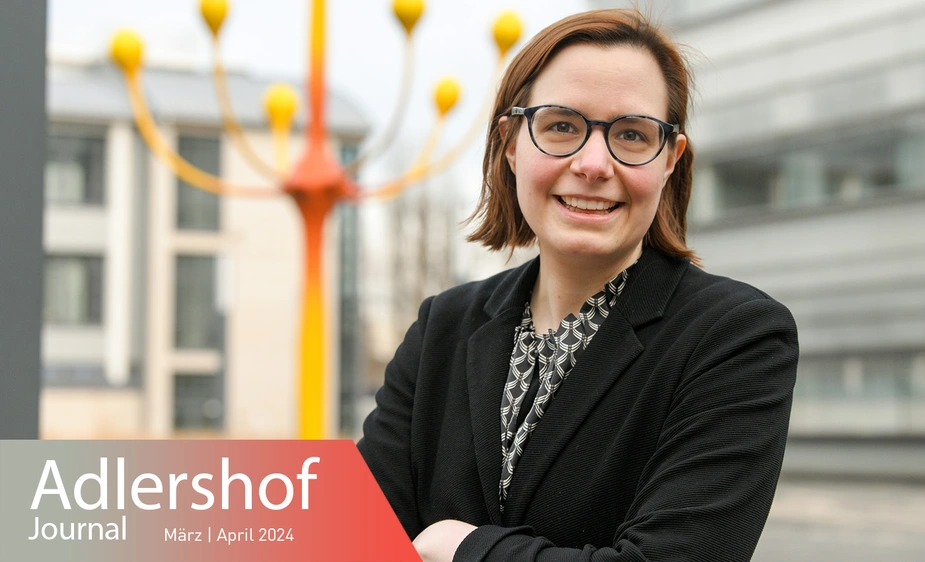
Using simulation and machine learning, Janine George is on the hunt for more efficient materials to advance the transition to clean energy, or Energiewende. Apart from saving her a lot of time, it helps her find new types that scientists had not yet considered. The energy transition often seems limited to questions like: What sources does electricity come from? Or how much greenhouse gas is involved? However, it also depends crucially on materials. The more efficient solar cells or batteries work, for example, the less energy is needed to power a modern society.
Janine George is working to discover new, better materials. She is not doing so by applying the trial-and-error method in a laboratory but based on computational methods. “We use calculations from quantum mechanics and methods of machine learning to predict the properties of materials and to develop new materials for certain applications in a very targeted way,” she says, as head of the junior research group Computational Materials Design at the Federal Institute for Materials Research and Testing, or BAM. “First, we create large databases containing information on potential materials without having to synthesise and experimentally characterise all these materials.” This saves a lot of time. They can be used to calculate data for idealised crystals, for example, like the ones used in the more efficient solar cells of the future. These data are easily comparable. When experimenting with real samples in a lab, however, defects in the crystal lattices result in problems and no two conditions are ever exactly the same. “With the help of these methods, we can accelerate materials development by years, if not decades.”
She has been enthusiastic about so-called computational chemistry since studying at RWTH Aachen University, says the 34-year-old, and about the hope of finding new materials much more rapidly. She came to BAM in 2021 after completing her PhD in Aachen, a post-doc stint at the Université Catholique de Louvain in Belgium, and a research stay at Oxford. At BAM, the prospects of AI-based materials research had already been recognised and George received a permanent position and the funding to set up a junior research group.
George benefits from Berlin’s research landscape, particularly when applying for third-party funding. Besides geography, however, she doesn’t like to narrow her focus. The six, sometimes eight-strong team of scientists from chemistry, physics, and materials science is international by design—one of the current PhD students is from Ecuador. George travels extensively, including now more often to Jena. She was made professor of computational materials science at the city’s Friedrich-Schiller-Universität.
In December, she received an award as a junior researcher by the Foundation Werner-von-Siemens-Ring. “Through her work, information from the analysis of chemical bonds can be combined with other material properties on a large scale and used to test chemical rules or derive new ones, and, in addition, to develop models for material properties based on machine learning,” according to the jury's citation. “With her work, she is making an important contribution to the energy transition.”
Teaching, research, leading a team – how does she do it? George hesitates for a short moment. “Yes, it sounds like a lot, but it’s all quite manageable when applying good time management.” One of the more recent subjects is thermoelectrics. This involves metals and semiconductor compounds that transform heat into electricity. This has been a topic of research for decades because the effect promises an elegant way of using waste heat, which would otherwise evaporate into the environment. Thermoelectrics have been used in astronautics for a long time. However, there is yet to be a major breakthrough in everyday applications.
“We hope to find better materials using our methods,” says George. The researchers use supercomputers and AI to calculate things like thermal conductivity and oscillatory behaviour, among others. “Our approach is significantly faster if materials are synthesised and individually tested first,” says George with conviction. “Especially material classes that haven’t yet received proper attention can be identified faster using a data-driven approach.” Another hopeful step towards a successful application.
Ralf Nestler for Adlershof Journal
BAM interview: “Data-driven science to discover new materials for safe applications”